Go behind-the-scenes of our latest project with the Bennett Institute for Public Policy at the University of Cambridge and learn more about how we’re using Real-Time Industrial Classifications to measure supply chains and explore the relatedness of key sectors in East Anglia.
Sector relatedness
The current economy is transforming the way we think about sectors, the way sectors interrelate, and the resulting supply chains. Research in technological relatedness and sectoral relatedness shed light on this context. Work in this field shows a movement towards tighter connections between sectors in the modern economy.
These findings are having a big effect on the way we understand supply chains. Nowadays, the provision of a product or a service does not always depend on a linear or sequential supply chain. Instead, supply chains look more like networks: companies tend to participate in more than one way in the production and provision of services and operate in more than one field at the same time. It is important to capture, access, and analyse data that illustrates this change.
Working with the Bennett Institute
In this project, we are exploring the concept of relatedness for 7 emergent economic sectors in the region of East Anglia.
The research has been designed and conducted in partnership with the Bennett Institute, which leads the Productivity Institute‘s Cambridge Hub. The Bennett Institute’s work is critical to understand the performance of the regional economy, creating knowledge that shapes policymaking.
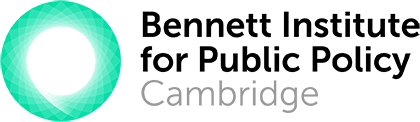
The Bennett Institute is interested in exploring relatedness across sectors in East Anglia, paying special attention to the role of enabling technologies such as Artificial Intelligence. We look into this by applying network analysis to Real-Time Industrial Classifications (RTICs), which allows us to quantify and visualise the links between sectors. This type of input can become a central resource to explore industrial relatedness and the potential for knowledge and skills transfer.
Our methodology
RTICs are an efficient method not only to capture companies in emergent sectors but to articulate them considering their role in supply chains, the technologies they use, or the final product/service they offer. RTICs are built with the machine learning function of The Data Explorer, which processes the website text data for 1.6 million companies.
Website examples of the sector of interest are fed into the platform, allowing the algorithm to identify the sector’s relevant language. Then, examples of company websites outside of the sector of interest are provided. This input allows the platform to create a framework for industrial classification based on the website text data, which is scored against all other available websites. The output is a list of companies whose websites meet the industrial classification framework’s criteria.
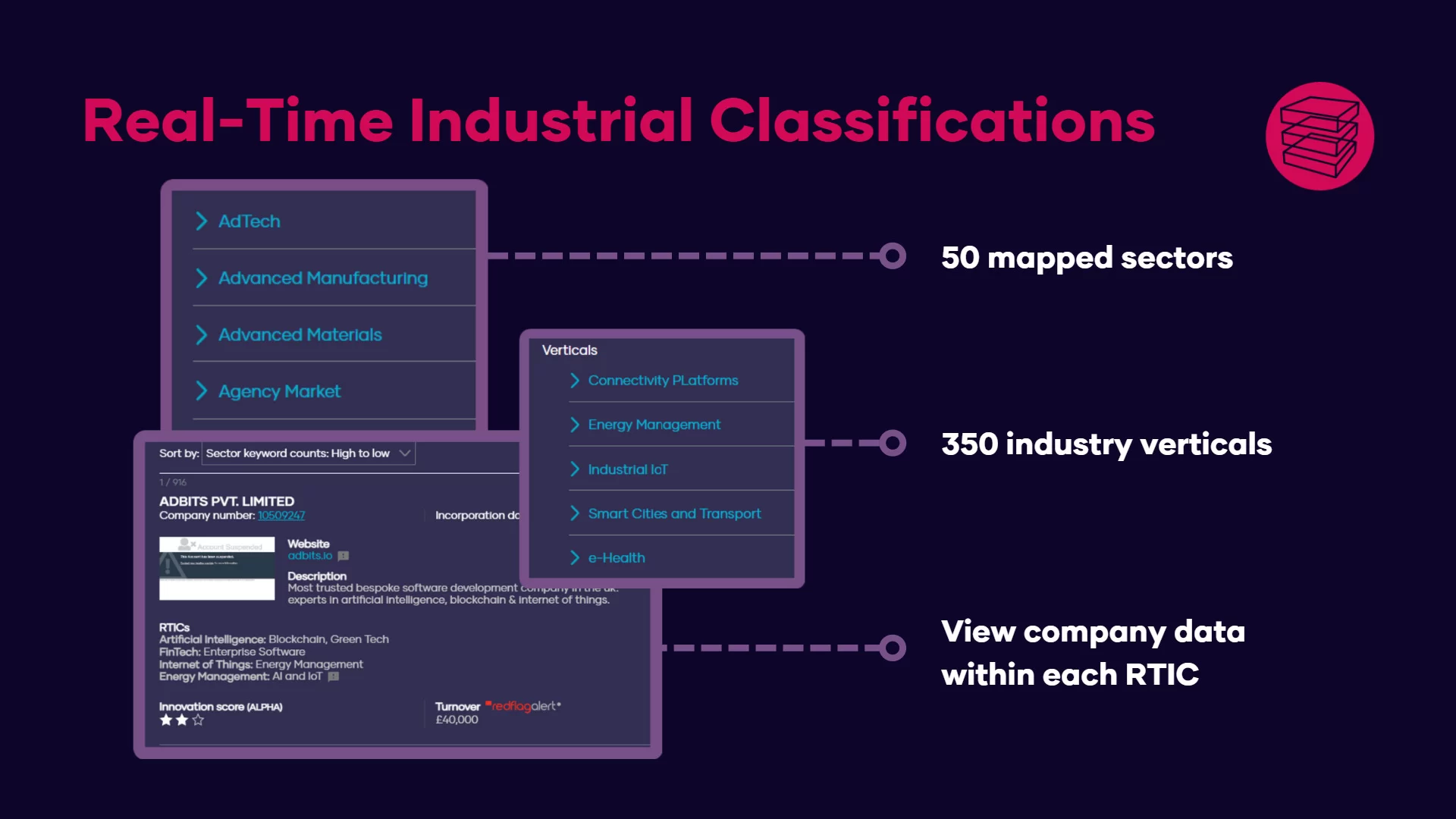
This exercise is repeated for the different segments of activity that build up a sector. The process of selecting the subcategories (or industry verticals) that make up a sector results in taxonomies for industrial classification.
This process introduces how RTICs allow investigating sectoral relatedness. RTIC classifications are not categorical and mutually exclusive, meaning that a company can be classified in more than one sector or industry vertical at the same time. For example, if a company develops Internet of Things (IoT) products that use proprietary Artificial Intelligence technology, this company will be classified both in the IoT and Artificial Intelligence RTICs.
Hence, the RTIC approach represents Industrial sectors relationally, building an understanding of the overlap across industry verticals and RTICs. Being able to quantify the number of companies classified into two or more groups at the same time suggests an economic, technological, and/or social collaboration between two sectors (RTICs) or supply chain activities (industry verticals).
RTICs and network analysis: building supply networks
There is general agreement in that technology transformations have made some supply chains more fluid and flexible, moving away from linear production processes. For example, several sectors may use the same technology for different purposes, creating bridges between traditionally unrelated sectors. This characteristic is making it progressively more difficult to map supply chains. To tackle this issue, we suggest the application of network analysis to RTIC datasets.
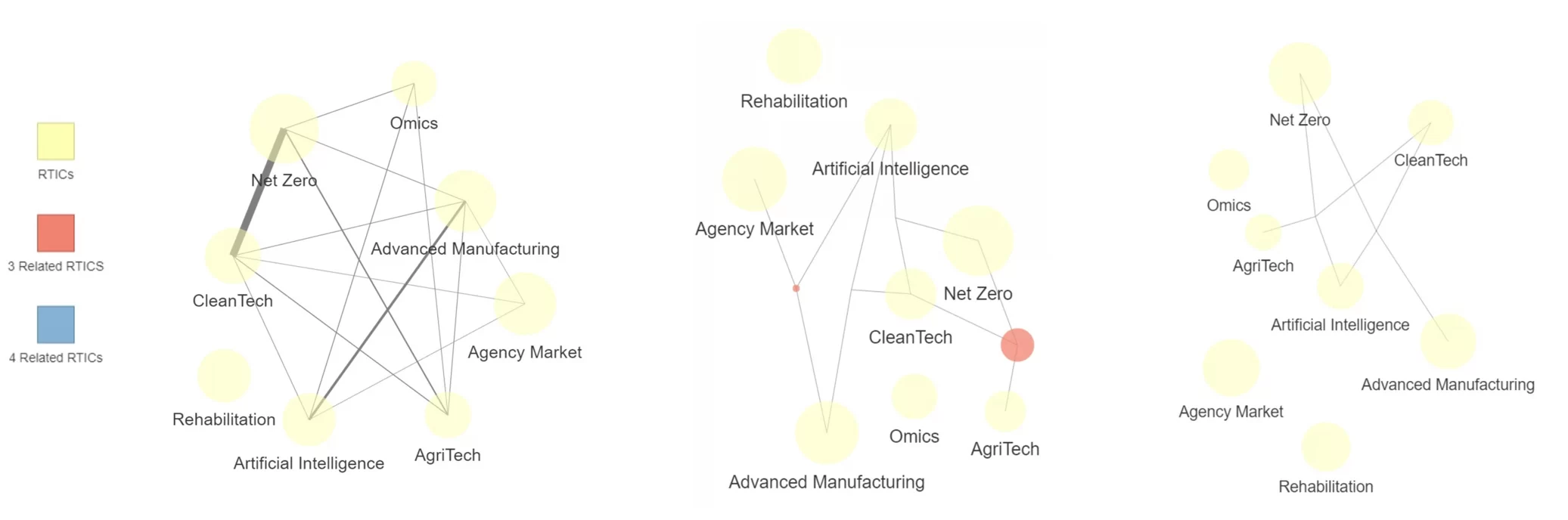
Network analysis has been applied to economic research for a considerable time. However, it has not been specifically applied to modelling supply chains, technological relatedness or evidence to investigate knowledge transfer. The data structure of RTICs allows the application of network analysis methods, shedding light on sectoral relatedness and newer supply chain structures.
Network analysis interrogates the relationships amongst different nodes. In this case, each node represents a sector or industry vertical, while the links quantify the number of companies that have been classified in more than one of these at the same time. This way, we can measure the density of the network, identify hierarchal structures and find points of encounter between seemingly unrelated nodes.
What’s next?
Working with the Bennett Institute we will apply this method to the business population of 7 RTICs based in East Anglia. The aim is to understand whether traditionally strong sectors in the region, like segments of Life Sciences industries and marketing, relate to each other through enabling technologies such as Artificial Intelligence.
Interested in the outcome? We’ll look to post more about our findings and insight from the project over the next few months.
In the meantime, if you’d like to learn more about our platform or have your own project you’d like to discuss with us, please feel free to contact us today.