Learn about how we worked alongside Innovate UK KTN (IUK KTN) to define the AI taxonomy in this deep dive article written by Dr. Fatima Garcia (The Data City) and Dr. Caroline Chibelushi (Innovate UK KTN).
When we think of artificial intelligence (AI) there is a tendency to focus on advanced machine learning algorithms, deep neural networks, or computational cybernetics. AI is a technology which is making an impact on everything around us. However, AI taxonomy plays a very important role in bringing AI to life. If we consider AI as a disruptive technology that will help us to achieve our UK AI strategy visions, it is necessary to create scenarios and identify the most relevant use cases within each scenario. AI taxonomy has the capability to extract and connect use cases with technology, regulations, skill requirements, and much more. When applied in different environments, it will monitor and track our abilities. If appropriately developed, an AI taxonomy can serve as a backbone for a vision driven, accelerated approach to reaching the UK AI strategy objectives of becoming a world leader in AI.
In this paper, we introduce the AI taxonomy formed by Innovate UK KTN and later adopted and extended by The Data City. Beyond the AI taxonomy extension, The Data City have used the taxonomy to develop Real-Time Industrial Classification (RTIC) to capture data from various databases, web crawling and applying machine learning techniques. RTIC is a methodology that uses website text as the main criteria for industrial classification, grouping companies according to common language patterns. The result is a database generated by training the machine learning technology with the taxonomy input. AI taxonomy in RTIC ensures a consistent approach to AI technologies and corresponding application scenarios. The results of the RTIC approach to utilising AI taxonomy, were used successfully to support the Department of Culture Media and Sport in its policy-making activities.
Background
A combination of knowledge and skills in AI, and experience in supporting AI suppliers and consumers, coupled with Innovate UK KTN’s responsibilities to support the government in delivering its policies led to the development of the AI taxonomy. Innovate UK KTN is a public sector organisation that exists to connect innovators with new partners and new opportunities beyond their existing thinking – accelerating ambitious ideas into real-world solutions. Its main objective is to accelerate business innovation and growth, so as to ensure that the UK economy is healthy and sustainable.
The Innovate UK KTN AI expert Dr. Caroline Chibelushi used her experience to design an AI taxonomy which allows the mapping of the AI ecosystem in the UK. The design is based on the expert’s vision that AI effects ecosystems as well as society, and that AI-specific measures will always require context. Innovate UK KTN perceive AI technology as an enabler for a variety of applications, and the UK has an opportunity to lead in specific scenarios where AI is a central technology. Consequently, the taxonomy is constructed to reflect use cases and scenarios as starting points for any analysis with regards to AI. This perspective has not only supported us to deliver our business innovation and AI adoption responsibilities, but also help navigate and understand the UK AI ecosystem. In addition, the UK government has been enabled to make informed decisions on its policy development and delivery.
Taxonomy Development
The AI taxonomy was developed through 3 steps.
Step 1:
The Innovate UK KTN AI expert started to develop the taxonomy by firstly ensuring that AI and cybernetics are not used interchangeably. Although AI and Cybernetics are both based on the binary logic principle and human-machine interaction, the two concepts are different. AI is based on the idea of creating machines to mimic human intelligence and behaviour, whereas Cybernetics is the science of human-machine interaction that employs the principles, feedback, control and communication shown in Figure 1.
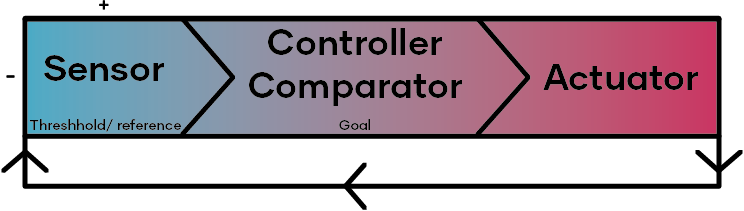
Based on this, we suggest that AI can be applied on cybernetics to enhance its performance. Also, the definition of cybernetics has helped the expert to identify cybernetics as shown in Figure 2.
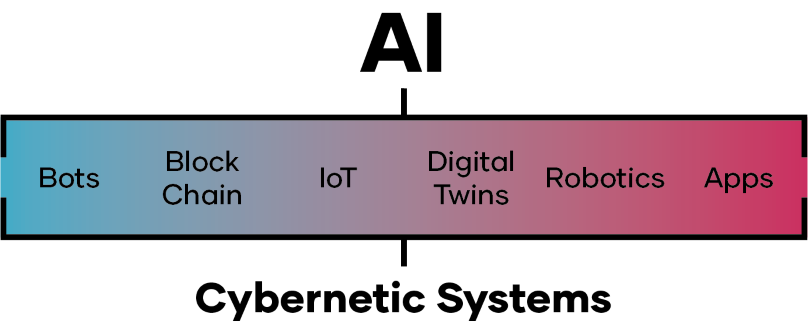
Bots, block chain, IoT, Digital Twins, robotics and apps are among the concepts within the cybernetic. These use AI in different ways, but they were not considered when developing the AI taxonomy because cybernetics is not AI.
Step 2:
This step is aimed at identifying the language that will define the AI taxonomy content. This language results from the use cases and scenarios experienced by the AI expert while supporting businesses in the UK, supplemented with literature review.
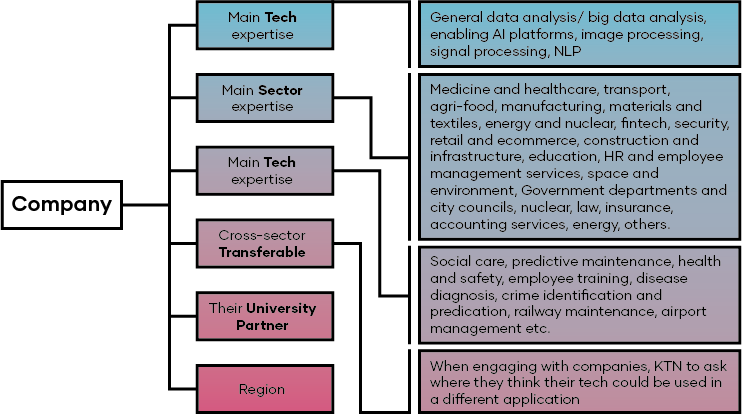
The sector scenarios shown in Figure 3 and the type of AI technologies which have been applied to the scenarios, fed into the taxonomy. For example, a company may offer an automated health and safety service to employees in transport sector (say rail and road repair or construction). Image processing and natural language processing (NLP) may be used to monitor if they are wearing correct personal protective equipment, while NLP can alert to dangers and actions that need to be taken before entering that work environment. Such a scenario will help to build a taxonomy that will allow identification of AI tools in the transport sector, AI tools in health and safety, and more.
Such scenarios resulted into the development of the Figure 4 taxonomy.
Data Analysis | Pattern recognition, correlations, outliers, prediction, optimisation, visualisation |
Enabling AI Platform | Pre-built algorithms and code frameworks |
Image Processing | Also known as Computer vision, Machine vision. Performing some operations on images in order to extract some useful |
Signal Processing | Audio processing example, Alexa, Chatbots, speech to text (real-time translation) |
Natural Language Processing | Text understanding, prediction, translation |
Cognitive Computing | Real time analysis of environment content and intent |
Data Manipulation | Data improvement, data integration, data modelling and simulation, real-time data transmission |
Figure 4 taxonomies assumed that the ML could be applied on all the 7 parts of this taxonomy.
Step 3:
The early version of taxonomy was submitted to The Data City to be refined and used to develop their Data Explorer tool. The early stages of refining the taxonomy were done in collaboration with the Dr. Chibelushi. Other activities were performed by The Data City as follows.
Applications of the AI taxonomy in RTIC methodology
The AI taxonomy was the first original taxonomy applied to the production of RTICs. The exercise had a significant impact on the RTIC method overall, helping set the basis for the definition of a novel process to produce industrial data. The RTIC method for industrial data capture has been used by public sector organisations like DCMS or BEIS, academic institutions like Edinburgh University and the Bennett Institute, and key knowledge transfer organisations like Innovate UK KTN or the Catapults. Hence, the AI taxonomy together and the RTIC methodology are having an increasing role in evidence-based decision-making in the UK.
In this section, we discuss the practical applications of AI taxonomy and RTIC methodology. We specifically highlight two projects where critical policymaking and networking organisations, DCMS, the Bennett Institute or Midlands Engine, used the AI taxonomy and the subsequent RTIC. Then, we compare the RTIC methodology with other website text methodologies for industrial classification and other available datasets. Comparatively, RTICs assure a correct match between companies as legal entities and their websites, expanding the scope of traditional industrial classifications. We finish by introducing the limitations of this approach and with a call for a unified effort in keeping industrial taxonomies updated and representative.
DCMS: finding promising and critical UK companies across high-tech sectors
The Department for Digital, Culture, Media and Sport was interested in identifying critical and promising companies working in the UK. Innovate UK KTN and The Data City partnered to produce a series of RTICs that makes it possible to find the targeted companies. Companies were labelled as promising and/or critical throughout the application of indexes over a large sample of financial variables.
DCMS expressed interest in the following emergent economic sectors: Artificial Intelligence, Data Infrastructure, Communications, Cyber Security and Cryptographic Authentication, Computer Hardware and Quantum Technologies. These economies are poorly represented in traditional frameworks for industrial classification, meaning that website text analysis and machine learning-based company classification pose as good alternatives for industrial data capture.
However, and as it has been argued previously, basing industrial classification in natural language processing techniques requires a taxonomical definition of the industries that considers language. The AI taxonomy was critical for this project not only because it was adopted by DMCS to map the AI sector in the UK, but because it set the basis for the production of taxonomies for the other sectors.
The successful completion of this project demonstrates the value of the AI taxonomy. It was acknowledged that the structure developed between Dr. Chibelushi and The Data City addressed the principal applications of AI technology and described the sector from a technology perspective. It yielded better results than other traditional and/or web-reading-based methodologies. This conclusion drew DCMS and IUK KTN to apply the same method to the rest of the sectors. Hence, this project demonstrates the value and impact of the AI taxonomy and the RTIC method for decision-making in the UK.
The outreach of the impact of this taxonomy and method can be appreciated by the increasing number of institutions engaging with it. For instance, the AI definition featured in this paper has been adopted in research projects with academic institutions such as the Bennett Institute, part of the Productivity Institute. The Bennett Institute was interested in exploring relatedness across several technological and service-based sectors in the region of East Anglia. Understanding these relationships would facilitate understanding the potential for knowledge and technology transfer between seemingly unrelated industries.
Regional organisations directly involved in economic development have consistently used the AI RTIC to understand the sector too. For instance, Midlands Engine and Northeast LEP selected the AI RTIC to understand the performance of their region against others and in relation to the UK overall. This information is central for regional organisations to inform policymaking, support the local business base and encourage networking, exploiting the benefits of evidence-based decision-making.
Ultimately, it is important to note that the increasing use of this definition by different types of organisations can help normalise the view of the sector. A unified approach to Artificial Intelligence makes possible a cohesive view of the sector across regions and scales, facilitating data sharing and communication between agencies.
The RTIC method: a comparison to other industrial classification approaches.
Traditional frameworks of industrial classification, like SICs and NAICS, continue to have a central role in economic policy. However, rapid change in economic conditions and technological developments has prompted some institutions to investigate new methods of data capture. The reason behind this is a need for data representative of industries poorly defined by obsolete frameworks for industrial classification. In this section, we discuss the RTIC methodology and compare it with other existing methods for industrial classification, arguing that it conveys an accurate view of the market. We first explore the benefits of RTICs before SICs and then move on to compare the approach to language-based classification with other methodologies. Altogether, this commentary will justify the application of the AI taxonomy with the RTIC method and not others.
The benefits of using the AI taxonomy and the RTIC methodology before SICs are based on the way the two approaches tackle industrial classification. On one hand, SICs are a categorical framework that requires companies to classify themselves using a preconceived list of coded economic activities. Hence, companies need to choose codes that describe their activity, and they are later grouped according to the codes they selected. SICs are used internationally, meaning that different countries around the globe use them to classify economic activity. This has great benefits, as far as it supports international comparisons and integrated action. However, they were last updated in 2007. This has relevant implications for sectors like Artificial Intelligence or quantum technologies, which do not have an equivalent SIC. In this situation, companies need to find the nearest match to their economic activity, leading to increasing numbers of companies classified in the “Other” categories within the scheme. This context makes it very difficult to explore the size a value of a sector or economic activity that is not directly represented by a SIC, setting boundaries for the sectors that can be directly interrogated.
On the other hand, RTICs offer a way out of this situation by using language as the main criteria for industrial classification. The Data City have been able to correctly match almost 1.6 million companies -legal entities reported active as per Companies House- to their correct URL. Then, they capture website text data thanks to web-crawling technology, building a database that links companies -and all their related financial, location and contact information- with text information that describes their activity and products. The companies’ website text is what drives industrial classifications and the production of RTICs and it is used to train the algorithm into grouping similar companies.
The fact that RTICs use language as criteria for industrial classification builds a flexible and adaptable methodology that represents the changes in the industry. As companies update their websites, RTIC methodology makes visible trend changes, the evolution of processes or products and the transformation of dominant practices. In contrast, SICs or other rigid frameworks for industrial classifications do not capture those changes in the economic field, limiting the visibility of newer industries and technologies.
RTICs are not the only method for industrial classification that uses website text. There are other available approaches that capture websites and analyse the concepts used as means to identify common products and/or services. However, these methodologies normally scan the whole of the web and do not limit their website text data capture to legal entities active as per Companies House. This means that some of the websites captured do not directly represent a real and/or active economic entity, creating issues when sizing and valuing a sector.
Altogether, RTICs make it possible to define an industry considering the way companies describe themselves in their website text while making sure that active economic actors are included. It implies that has the benefits of an SIC-based approach -there is available data at the company level that can be aggregated into economic sectors- and the self-updating and highly representative qualities of text-based industrial classification.
Limitations
No method is perfect, and this novel approach for industrial taxonomies and data has a series of caveats that is important to introduce. We will concentrate on two main problematics: on one hand, the accuracy of the company-website match; on the other hand, bias in identifying the relevant terminology during the taxonomy production process. These two pose significant risks to industrial classification, however, technological developments and user participation continue to offer solutions and alternatives.
Considering the approach for data capture used by the RTIC method, if a company has not been correctly matched to a website -or the website text cannot be scraped-, it will lack text data and it the algorithm will not be able to classify it in any group. Similarly, if a company was matched to the wrong URL, it could be classified in the wrong sector. This point highlights the importance of accurate URL matching for the accuracy of RTICs. Currently, the URL matching accuracy is over 95% and the framework for URL matching continues to be updated and improved.
Regarding the process of taxonomy production, it is foremost important to admit that previous bias and views of the sectors can cap the scope of the taxonomy. The taxonomy identifies the pockets of activity that share language patterns and engage in similar activity, recording common keywords and key phrases that can be used to identify relevant companies and train the algorithm to find others that share language patterns. Therefore, failing to identify a particular activity, narrative, practice or product would lead to the exclusion of that type of companies from the algorithmic search. This means that the affected companies would not be classified in the corresponding sector and the sector would be presented in narrower terms.
Nonetheless, the frequently updating nature of RTICs and the review and collaboration of different institutions in the definition of industrial sectors are proving to be effective strategies to minimise these issues. The underlying method for URL matching is in constant development while user input and reported wrong matches help identify the edge cases and abnormalities. Altogether, these efforts have shown to produce great improvements in the overall RTIC method, which gives consistent and confider views of the size, value and geographic distribution of economic sectors like AI.
Conclusions
The different applications of the AI taxonomy and the RTIC method demonstrate the robustness of the approach. They not only yield accurate results, but they make it possible to track the development of the sector. Using language as criteria for industrial classification allows for the progressive and periodic updating of a sector’s definition. This is translated into machine-learning-based techniques that classify companies according to common language patterns, automating part of the process of sector data updating. This is a critical feature to capture data on the ever evolving and rapidly changing economic conditions, and more for newer technology sectors such as Artificial Intelligence.
It is also important to stress that the production of the AI taxonomy was a critical step for the definition of RTIC methodology. The production of a dataset that successfully targeted companies working in AI allowed The Data City to replicate the same methodology for other sectors. This represents a key step forward for economic data capture and industrial classification methods, creating an alternative approach to represent industries and determine their size and value.
Ultimately, the fact that RTICs have been consistently used by organisations in the public and private sectors as well as by academia and accelerators – such as the Catapults – conveys an important message. RTICs are increasingly becoming an asset for evidence-based decision-making and having an influence over political and economic decisions that affect populations and territories. This is why providing background on the process of RTIC production is necessary for other actors to understand how RTICs fill a gap in industrial data availability. Likewise, it constitutes an opportunity to call for an open discussion on industrial classification, sector definitions and data updating that sets the basis for an approach to data co-production that engages sector experts, relevant institutions and the public.
This article was written by:
Dr. Fatima Garcia
Dr. Caroline Chibelushi